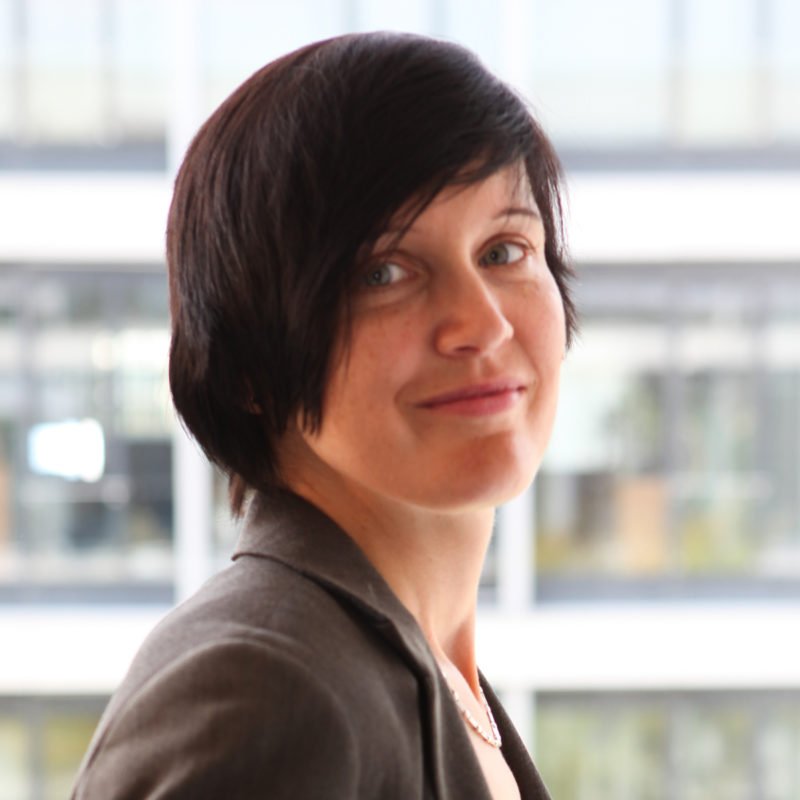
Speakers:
Obtaining Uncertainty Estimates from Neural Networks Using TensorFlow Probability
Date:
November 18, 2019
Time:
4:00 pm
Room:
Estrelsaal C1 & C2
Summary:
Among data scientists, there is hardly a need to stress the importance of uncertainty estimates accompanying model predictions. However in deep learning, successful though it may be, there is no straightforward way to assess uncertainty. As of today, the most promising approaches to modeling uncertainty are rooted in the Bayesian paradigm. Commonly in that paradigm, we distinguish between aleatoric (data-dependent) and epistemic (model-dependent) uncertainty. In this session, Sigrid will show how both can be modeled blending deep learning (TensorFlow) and probabilistic (TF Probability) software. All demo code will be run using tfprobability, the R wrapper to TensorFlow Probability.