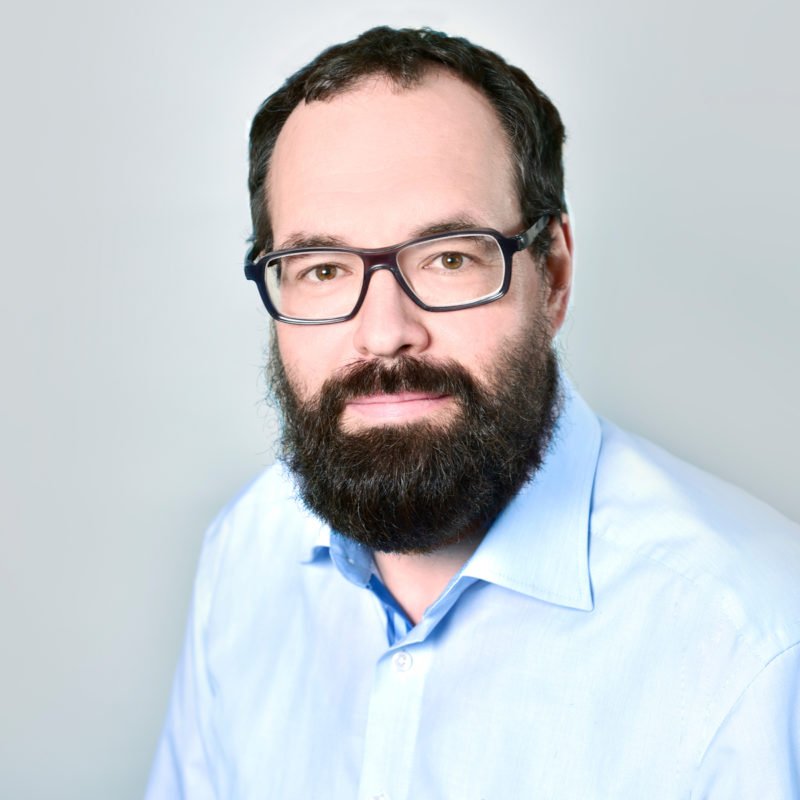
Speakers:
Model-agnostic Black-Box Enlightening with SHAP
Date:
November 16, 2020
Time:
1:00 pm
Summary:
The acceptance of machine learning algorithms in predictive analytics heavily depends on their interpretability. With the growing complexity of deep learning and machine learning models, the need for explanability techniques is continually increasing. Model-agnostic explanability techniques are a topic of on-going research in the field of explainable AI, since they allow to compare different modeling approaches within one concept. This deep dive presents SHAP, a model-agnostic game theoretic approach. SHAP unifies existing concepts like LIME and Shapley regression. State-of-the-art software implementation, highly-tuned algorithms for tree-based and deep learning models, as well as unified explanations of both global and local feature importance made SHAP the new gold standard in the field of XAI. The session will demonstrate the advantages and elegance of SHAP in the field of predictive analytics, and show why it belongs in every data scientist’s toolbox.