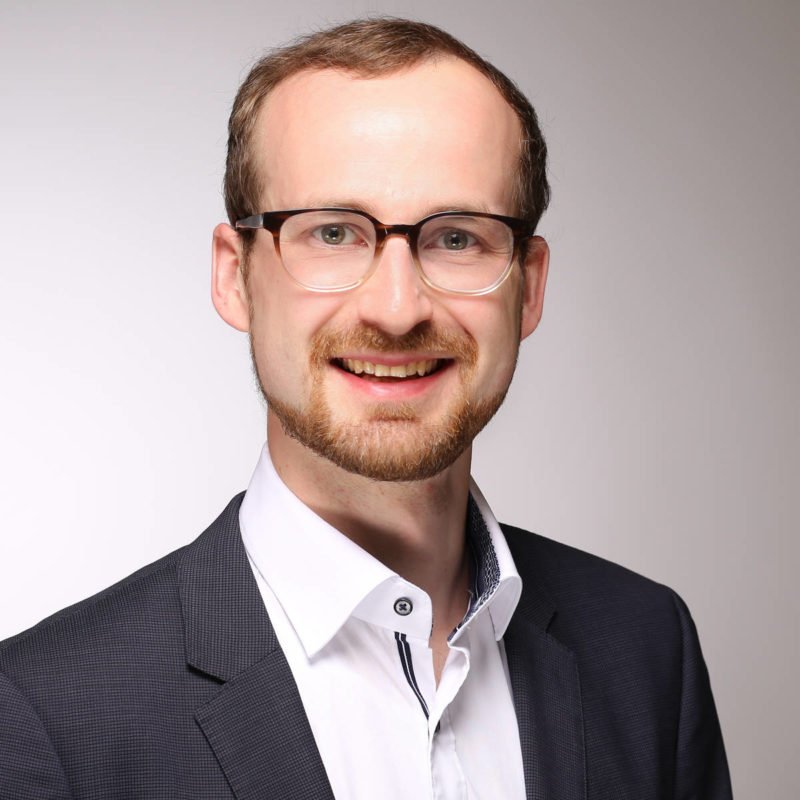
Sprecher*innen:
Stock Price Prediction and Portfolio Optimization Using Recurrent Neural Networks and Autoencoders
Datum:
Montag, 16. November 2020
Zeit:
13:00
Summary:
Financial time series forecasting is a challenging problem. Deep learning approaches, such as recurrent neural networks (RNNs), have proven powerful in modelling the volatility of financial stocks and other assets, as they are able to capture non-linearities in sequential data. Recent studies have shown that RNNs have surpassed well-known autoregressive forecasting models (Siami-Namini, 2018). Besides forecasting the next value of a stock, creating an optimal portfolio is equally important. Deep portfolio theory (Heaton et.al.,2018) uses autoencoders to model the non-linearity of the time series to accurately predict returns. Within this session, Julian will extend this approach by first performing a 10-day ahead forecast and then train an autoencoder model to construct an ideal portfolio that incorporates previous and future stock market information. You will be provided with a theoretical understanding of how RNNs and autoencoders work and how to apply them on multivariate timeseries forecasting and portfolio optimization problems. The new model is then applied on financial stock data and compared to traditional portfolio optimization methods. Finally, the session will end with presenting an overview of key challenges and current research topics within that field.